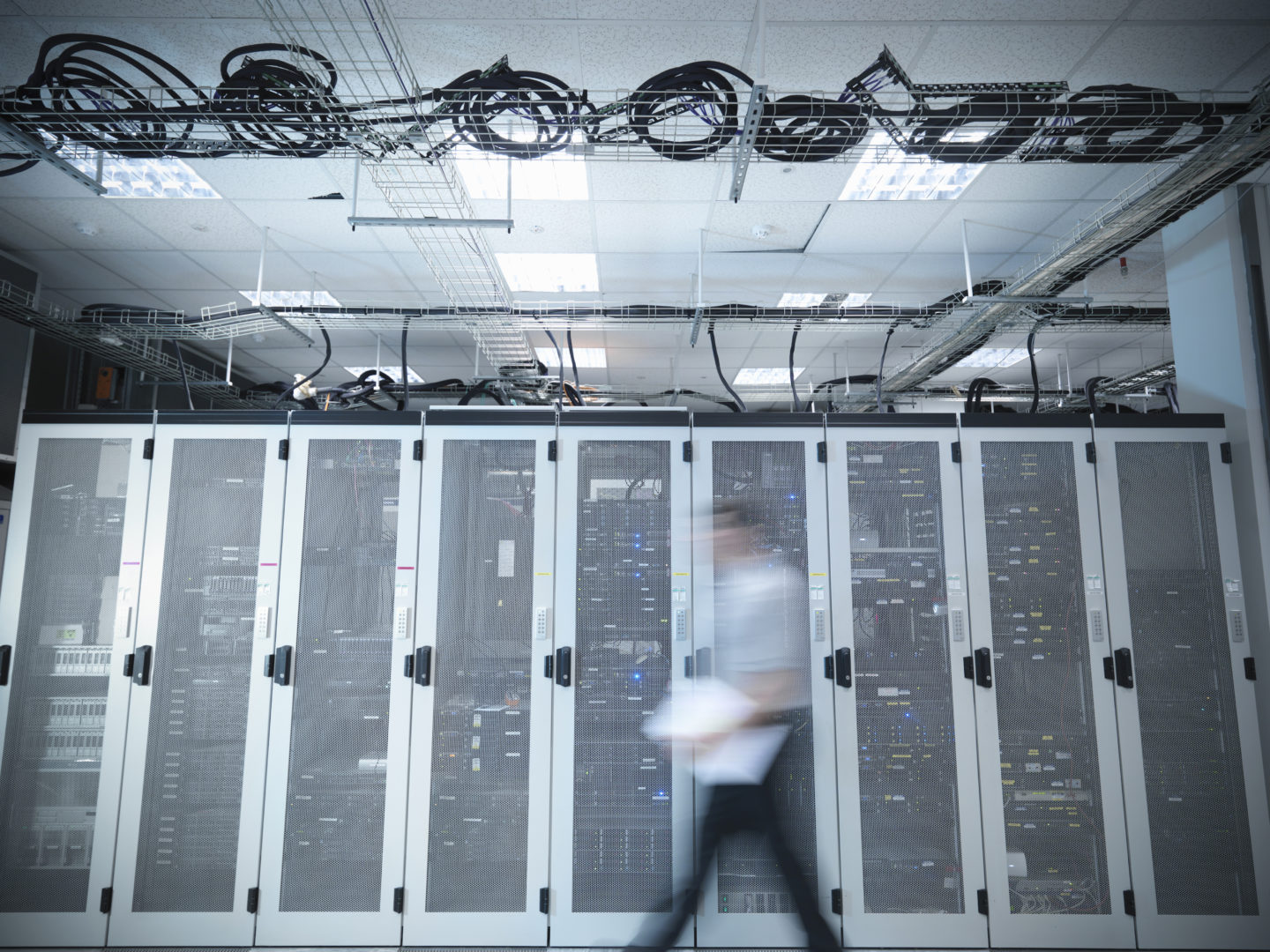
My father passed away recently, and so I’ve found myself in the midst of a cycle of grief. And, in thinking about good blog topics, I realized that many of the organizations I’ve worked with over the years have gone through something very much like grief as they’ve come to confront big data challenges …and the stages they go through even map pretty cleanly to the five stages of grief! So this series was born.
So it’ll focus on the five stages of grief: denial, anger, bargaining, depression, and acceptance. I’ll explore the ways in which organizations experience each of these phases when confronting the challenges of big data, and also present strategies for coping with these challenges and coming to terms with big data grief.
Part One: Denial
”We don;t have a big data problem. Our Oracle DBA says so.”
Big data is a stealth tsunami—it’s snuck up on many businesses and markets worldwide. As a result, they often believe initially that that they don’t need to change. In other words, they are in denial. In this post, I’ll discuss various forms of denial, and recommend strategies for moving forward.
Here are the three types of organizational “denial” that we’ve seen most frequently:
They don’t know what theyre missing
Typically, these organizations are aware that there’s now much more data available to them, but don’t see that how it represents opportunity to their business. Organizations may have listened to vendors, who often focus their message on use cases they want to sell into—which may not be the problem a business needs to solve. But it’s also common for an organization settle into its comfort zone; the business is doing just fine and the competition doesn’t seem to be gaining any serious ground. So, the reasoning goes, why change?
The truth is that, as much as those of us who work with it every day feel that there’s always a huge opportunity in big data, for many organizations it’s just not that important to them yet. They might know that every day, tens of thousands of people tweet about their brand, but they haven’t yet recognized the influence these tweets can have on their business. And they may not have any inkling that those tweets can be signals of intent—intent to purchase, intent to churn, etc.
They don’t think its worth doing
Organizations in denial may also question whether dealing with big data is worth doing. An organization might already be paying a technology vendor $1 million or more per year for technology… and this to handle just a few terabytes of data. When the team looks at a request to suddenly deal with multiple petabytes of data, it automatically assumes that the costs would be prohibitive and shuts down that line of thinking. This attitude often goes hand-in-hand with the first item… after all, if it’s outrageously expensive to even consider a big data initiative, it seems there’s no point in researching it further since it can’t possibly provide a strong return on investment.
Somebody is in the bunker
While the prior two items pertained largely to management decisions based on return on investment for a big data project, this one is different. Early in my career I learned to program in the SAS analysis platform. As I pursued this for several different firms, I observed that organizations would tend to build a team of SAS gurus who held the keys to this somewhat exotic kingdom. Key business data existed only in SAS datasets which were difficult to access from other systems. Also, programming in SAS required a specialized skillset that only a few possessed. Key business logic such as predictive models, data transformations, business metric calculations, etc. were all locked away in a large library of SAS programs. I’ve spoken with more than one organization who tells me that they’ve got a hundred thousand (or more!) SAS datasets, and several times that many SAS programs floating around their business… many of which contain key business logic and information. As a result, the SAS team often held a good position in the organizational food chain, and its members were well paid.
One day, folks began to discover that they could download other tools that did very similar things, didn’t care where the data resided, cost a fraction of SAS, and required less exotic programming skills.
Can you see where this is going?
I also spent some years as an Oracle DBA and database architect, and witnessed very similar situations. It’s not uncommon—especially given how disruptive big data technologies can be—to see teams go ”into the bunker” and be very reluctant to change. Why would they volunteer to give up their position, influence, and perks? And so we now are at the intersection of information technology and a classic change management challenge.
Moving forward past denial
For an organization, working through the denial stage can seem daunting, but it’s very do-able. Here are some recommendations to get started:
Be prepared to throw out old assumptions. The world is rapidly becoming a much more instrumented place, so there are possibilities today that literally didn’t exist ten years ago. The same will be true in another ten years (or less). This represents both opportunity and competitive threat. Not only might your current competitors leverage data in new ways, but entirely new classes of products may appear quickly that will change everything. For example, consider the sudden emergence in recent years of smartphones, tablets, Facebook, and Uber. In their respective domains, they’ve caused entire industries to churn. So it’s important to cast a broad net in terms of looking for big data projects to deliver value for your business.
Big data means not having to say ”no”. I’ve worked with numerous organizations who have had to maintain a high cost infrastructure for so long that theyre used to saying “no” when they’re approached for a new project. And they add an exclamation point (”no!”) when they’re approached with a big data project. Newer technologies and delivery models offer the chance to put much more in the hands of users. So, while saying “no” may sometimes be inevitable, it no longer needs to be an automatic response. When it comes to an organization’s IT culture, be ready to challenge the common wisdom about team organization, project evaluation, and service delivery. The old models—the IT service desk, the dedicated analyst/BI team, organizing a technology team into technology centric silos such as the DBA team, etc. may no longer be a fit.
Big data is in the eye of the beholder. Just because vendors love to talk about Twitter (and I’m guilty of that too), doesnt mean that Twitter is relevant to your business. Maybe you manufacture a hundred pieces of very complex equipment every year and sell them to a handful of very large companies. In this case, it’s probably best not worry overmuch about tweets. You might have a very different big data problem. For instance, you may need to evaluate data from your last generation of devices which had ten sensors that generate ten rows of data per second each. And, you know that the next generation will have ten thousand sensors generating a hundred rows per second each—so very soon it’ll be necessary to cope with around ten thousand times as much data (or more—the new sensors may provide a lot more information than the older ones). And if the device goes awry, your customer might lose a $100 million manufacturing run. So don’t dismiss the possibilities in big data just because your vendor doesn’t talk about your business. Push them to help you solve your problems, and the vendors worth partnering with will work with you to do this.
Data expertise is a good thing. Just because you might not need ten Oracle DBAs in the new world doesn’t mean that you should lay eight of them off. The folks who have been working intimately with the data in the bunker often have very deep knowledge of the data. They frequently can retool and, in fact, find themselves having a lot more fun delivering insights and helping the business. It may be important to re-think the role of the ”data gurus” in the new world. In fact, I’d contend that this is where you may find some of your best data scientists.
While organizational denial is a tough place to be when it comes to big data, it happens often. And many are able to move past it. Sometimes voluntarily, and sometimes not—as I’ll describe in the next installment. So stay tuned!
Next up:
Anger: ”We missed our numbers last quarter because we have a big data problem! What the heck are we going to do about it?”